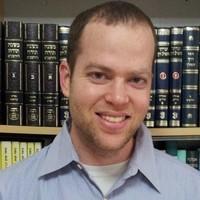
Spectral methods for unsupervised ensemble learning and latent variable models
The use of latent variables in probabilistic modeling is a standard approach in numerous data analysis applications. In recent years, there has been a surge of interest in spectral methods for latent variable models, where inference is done by analyzing the lower order moments of the observed data. In contrast to iterative approaches such as the EM algorithm, under appropriate conditions spectral methods are guaranteed to converge to the true model parameters given enough data samples.
The focus of the seminar is the development of novel spectral based methods for two problems in statistical machine learning. In the first part, we address unsupervised ensemble learning, where one obtains predictions from different sources or classiers, yet without knowing the reliability and expertise of each source, and with no labeled data to directly assess it. We develop algorithms to estimate the reliability of the classiers based on a common assumption that different classiers make statistically independent errors. In addition, we show how one can detect subsets of classiers that strongly violate the model of independent errors, in a fully unsupervised manner.
In the second part of the seminar we show how one can use spectral methods to learn the parameters of binary latent variable models. This model has many applications such as overlapping clustering and Gaussian-Bernoulli restricted Boltzmann machines. Our methods are based on computing the eigenvectors of both the second and third moments of the observed variables. For both problems, we show that spectral based methods can be applied effectively, achieving results that are state of the art in various problems in computational biology and population genetics.
Ariel Jaffe is a Gibbs assistant professor in the Applied Math program at Yale university. Ariel completed his PhD at the Weizmann institute of Science in Israel, under the guidance of Prof. Boaz Nadler. His main research interests include statistical machine learning and data analysis. He is also interested in applying machine learning methods to advance various applications in the field of computational biology. Prior and during his PhD studies, he led the research and development of the Duchifat project (2010-2017), where two Nano-satellites were developed and operated by teams of high school students. In 2014 he was chosen as an Israeli representative to the UN Outer Space Affairs. Between 2011-2013 he was with the CTO team in Alvarion, where he specialized in algorithms for indoor localization.